Four recommendations for bringing an AI product to market
Bringing an AI product to market successfully has proven more difficult than expected for many tech companies. These statistics illustrate the opportunity and the challenge.
- AI is projected to add $13 trillion to the global economy by 2030.
- However, a 2020 Gartner Inc. report found that in the previous two years, companies with artificial-intelligence experience moved just 53% of their AI proof of concepts into production.
Isurus’ perspective on AI-enabled solutions comes from multiple research engagements in the last three years. We work with a mix of growth-stage companies, and established companies adding AI to an existing product portfolio. We’ve provided insights on AI-enabled solutions in B2B sectors ranging from transportation to security to education. Across these diverse sectors and contexts, we see a common set of trends and implications for bringing an AI product to market.
Trends
The trends we’ve seen are the classic mix of good news and some bad news for bringing AI solutions to market.
Let’s start with the good news.
Data rich and insight poor
Businesses know their data is significantly underutilized. In the last 10 years, businesses got much better at collecting and monitoring metrics. Dashboards and other analytic tools proliferated. Yet, several major challenges remain. As analytics and reporting tools proliferated, so has the volume and type of data collected. The tools and skill sets present in any organization haven’t kept pace with the growth in data. Consequently, businesses recognize that they’re still dealing with the tip of the data iceberg. AI-enabled solutions resonate because they offer the potential to drive actionable insights out of an ever-growing mountain of data. Further, businesses recognize that they’ll face a competitive disadvantage if they lag peers in effectively harnessing AI.
Overcoming data silos
Many AI solutions address a frustration that persists in many companies –siloed data. Midsize and large companies collect and store data in a growing number of applications. They lack the capabilities to combine and analyze data across multiple streams. Yet, they understand the potential value of an integrated analysis that pulls data from many different sources. In the past, the solution required lengthy and expensive projects with unclear ROI. Buyers recognize the value of an AI solution that can combine data from multiple applications or sensors.
AI’s credibility challenge
Now for the bad news: AI-enabled solutions still face a credibility challenge. Across sectors, our research finds two main concerns about artificial intelligence. The first and broader concern is whether AI-enabled solutions will deliver results. Every early-stage technology faces this challenge and AI is no different. The nature of the concerns vary by sector and segment, and the application. The second concern about AI is the risk associated with flawed and biased algorithms. Most businesses are familiar with examples of biased algorithms through media coverage of high-profile problems. For example, Amazon reportedly scrapped an AI recruiting tool because it was biased against women. These issues have led a growing number of public companies to flag artificial intelligence as a risk in their annual reports.
Understanding the specific questions and concerns of the target audience is critical to bring an AI product to market successfully.
Recommendations for AI solution go-to-market strategy
Based on the trends in our research, Isurus has four recommendations for Sales and Marketing when bringing an AI product to market.
Show relevance and value
B2B buyers make meaningful investments in technology when it addresses a clear need. The typical mainstream B2B buyer won’t invest unless they’re convinced the solution offers meaningful improvement. To show relevance and value, our clients use market research to uncover buyer needs and pain points. Then, they apply these insights to develop the value proposition and messaging. For an AI product, the business outcome or benefits needs to take center stage in product messaging.
Align the vocabulary to the market
Most buyers have heard the various nomenclature associated with AI — machine learning, neural networks, deep learning and others. The terms may be familiar, but our research finds that these terms lack specific meaning or resonance with B2B buyers. Yet, many AI-enabled products continue to rely on these terms to communicate value or differentiation. To find the right vocabulary, start by exploring how the audience own words for these concepts – for example, how would they explain “artificial intelligence” to a colleague? Use these insights to select the key phrases and labels for use in marketing and sales. And remember behavioral economics Nobel laureate Daniel Kahneman’s advice that, “If you care about being thought credible and intelligent, do not use complex language where simpler language will do.” As AI-enabled solutions become more mainstream, the language and level of explanation required will change.
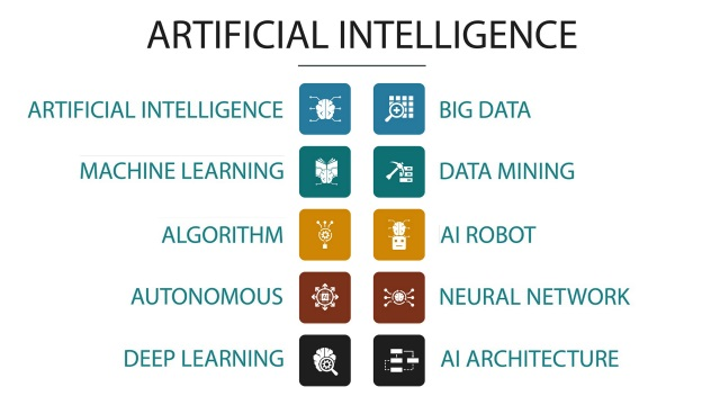
SecurityInfoWatch.com contributor Ray Bernard examined these terms in his ‘Real Words or Buzzwords?’ column.
Lower barriers to adoption
For the next several years, AI-enabled solutions will continue to be seen as new and, at least somewhat, risky. The degree of risk will vary by the solution, the sector, and other factors. The first step is to understand the nature and magnitude of the perceived risk. These insights can then be used to determine the appropriate risk-mitigating messages, content and sales processes. For some buyers, a comprehensive demo or white paper may be sufficient. Other buyers will require case studies or reference customer site visits. And in some sectors, a pilot will be required before they can fully commit to a solution.
Show that AI is accessible to the business user
A successful AI-enabled solution needs to address the “last mile” problem of adoption by the typical business user. Outside of large enterprises, most businesses lack data science and data analytics teams. They are not likely to adopt an AI-solution if they must add staff in order to realize value from the investment. Business buyers want to be confident the solution will provide value in the context of their existing talent and processes. The typical business user is not an analyst; they’re a manager tasked with making decisions based on the best information available and accessible to them. Go-to-market messages should communicate how the AI-solution facilitates better decisions in a typical user scenario.
The bottom line? Understand the buyer.
The common theme in these trends and recommendations is to understand the specific market and buyer, and incorporate these insights into the go-to-market strategy for an AI-enabled solution. From Isurus’ perspective, product marketers should conduct qualitative research to inform their strategy to bring an AI product to market. Qualitative insights provide more depth to uncover the business value drivers, barriers to adoption, and to find the right vocabulary for the sector. These interviews can be conducted with advisory board members, through informal research, or a more rigorous market research engagement.